What are the essential components of a Big Data Stack in 2023, and why are they important?
The modern world of data is a complex and ever-evolving landscape, with a myriad of tools and techniques available to organizations looking to make the most of their information. The key components of the modern data stack are data ingestion, data lakehouse, data transformation, data governance, BI, AI, and reverse ETL.
Data ingestion is the first step in any big data project. It involves bringing data from a variety of sources into a central database where it can be accessed and used. With so many different data sources, formats, and requirements, there are many ingestion tools available. The goal is to replicate raw data sources into the centralized data lakehouse consistently and reliably.
The data lakehouse is a powerful approach that combines the best aspects of a data lake and a data warehouse. Google Cloud BigQuery is a fully managed service that can handle structured, semi-structured, and unstructured data from multiple sources, even across public clouds. BigQuery supports SQL queries and provides powerful analytics tools, such as data visualization and machine learning capabilities.
After data has been ingested into the data lakehouse, the focus shifts to data transformation. This involves cleaning and validating the data to ensure its quality, and structuring it in a way that makes it usable for various purposes. Multiple layers of modelling techniques are applied, such as a normalized approach for enterprise data warehouse schemas and a denormalized data mart layer for BI and AI use cases. DBT or Dataform are used for the development and orchestration of these transformations.
Business intelligence (BI) is the process of extracting insights and value from data. Business users should have access to a self-service analytics environment with dashboards that help them make informed decisions. Additionally, AI and machine learning can bring even more insights into the data and make predictions for faster decision-making. Vertex AI of Google provides a range of services for implementing AI solutions, from custom models to fully managed APIs.
Reverse ETL is an emerging component of the modern data stack, where data is extracted from the data warehouse and integrated into third-party applications. This approach enables business users to leverage clean and valuable data in their day-to-day work within their tooling, such as marketing or sales platforms.
A crucial aspect of the modern data stack is data governance. This includes quality, security, privacy, discoverability, ownership, lineage, and lifecycle management of data. Proper data lineage is important to understand the full trajectory that data completes before it ends up as a KPI in a dashboard. Clear documentation on data assets and their definitions is essential for people to navigate the data and be aligned on business definitions. A data dictionary, data catalogue, and business glossary are used to store metadata on different levels of technicality. In larger organizations, assigning data owners and stewards facilitates the management and governance of different data assets in a distributed manner.
The modern data stack offers a wealth of tools and techniques to organizations looking to unlock the value of their data. By leveraging the power of data ingestion, data lakehouse, data transformation, data governance, BI, AI, and reverse ETL, businesses can gain powerful insights and make more informed decisions.
How has the landscape of Big Data tools changed in recent years, and what new tools are emerging as must-haves for a Big Data Stack?
Over the past years, we’ve witnessed a major shift in the world of data pipelines – from ETL to ELT. In the olden days, the norm was to extract data from the source, transform it in flight, and load it into the warehouse. But that’s not how things are done anymore. Now, we extract the raw data and load it as it is, and then perform the transformations in the lakehouse. And let me tell you, this approach has several advantages over the previous one!
With ELT, you get to do proper data validation between the source and the warehouse. Since the warehouse stores data in the same format as the source, it’s easy to check if the pipeline is running smoothly. On the other hand, transforming and storing data in a different format can cause some of the data elements to be lost or altered, making it difficult to use them in future cases.
Another benefit of the ELT approach is that it allows you to change transformations without losing your raw historical data. Third-party tools, like Dataform and DBT, have emerged to orchestrate SQL transformations within the warehouse, making the entire process even smoother.
Marketing teams have also come to realize the importance of leveraging data in their business processes. A composable customer data platform is now crucial to their success. The data lakehouse-centric approach is gaining more popularity, as it allows organizations to maintain a central place where the customer profile is being maintained.
Real-time data has become increasingly important, as people want to generate insights and act on them quickly. For example, personalized product recommendations on a website are based on past and current user behaviour. To handle streaming data, tools like Dataflow are being used to manage aggregations, low-quality data, and even late data.
Finally, Dataplex is an upcoming service that integrates nicely with the entire data stack. It automatically computes the data lineage of BigQuery tables based on the queries used to create them and provisions a data catalogue containing the standard metadata of those data assets. It also allows you to do quality checks, data profiling, and data loss prevention.
How can businesses ensure that their Big Data Stack is scalable and flexible enough to handle the ever-increasing volume and variety of data sources?
If businesses want to unlock the true potential of their operations and achieve unparalleled scalability and flexibility, the cloud is the way to go. It’s time to say goodbye to the days of managing your IT infrastructure and embracing the serverless architecture that reduces operational overhead and enables a scalable pay-as-you-go model.
My recommendation is to opt for a serverless approach wherever possible. With this approach, you can leverage powerful tools like Google Cloud Dataflow Prime for your data processing pipelines, which can scale horizontally based on the amount of data it has to process. This allows for vertical scaling of different steps in the pipeline to ensure that resources are used efficiently at every stage.
Without such a capability, resources may have to be overprovisioned for a large part of the pipeline if certain steps require more computing or memory. This would be inefficient and costly and could result in missed opportunities for growth and innovation. By embracing serverless architecture and cloud computing, businesses can achieve unrivalled agility, scalability, and efficiency in their operations.
What challenges can businesses expect to face when implementing and maintaining a Big Data Stack, and how can they overcome these challenges?
Every organization today aspires to become data-driven, but what does it take to achieve this goal? Simply put, it requires access to high-quality data that can inform strategic decision-making at every level of the organization. In the past, data was primarily managed by the technical department, but times have changed. We are now witnessing a shift towards a distributed data mesh approach, where data management, ownership, and stewardship are becoming decentralized to eliminate bottlenecks and increase adoption.
Each department is responsible for the quality of its data, but this decentralization can also introduce governance complexities. To overcome this challenge, it is still recommended to have a central governance layer to ensure that everyone uses the correct tools, frameworks, and practices, while also aligning on the global data strategy and prioritization within the organization. Creating this structure within an organization can be daunting, but at Devoteam G Cloud, we offer proposals on how to build a solid foundation for your platform. We work with your key stakeholders in a series of business and technical workshops to map out the platform and provide recommendations on the tools and processes needed to manage your data.
Our expertise helps you tackle the challenges of becoming a data-driven organization, including getting buy-in from the business side to make the necessary investments to build a future-proof platform. With our guidance, your organization can take the first steps towards becoming truly data-driven.
How can businesses leverage their Big Data Stack to drive insights and decision-making across the organization, and what best practices should they follow to maximize the value of their investment in Big Data tools?
In the past, we’ve often seen that when kicking off data projects, organizations start mapping all their data sources onto a very big data model. With this approach, they want to ingest all the data in a standardised data model into one central place, which means they’ll easily be working on a two-year project without actually bringing business value.
That’s why today we work on a more use-case-driven approach. In this approach, we first define what the use cases are that will benefit their business processes or that can bring value to their customers. We work more in an agile manner to be able to deliver those use cases. For example, we do inspirational sessions on how ML and AI can boost their business or what kind of BI dashboards and self-service analytics requirements have the most value within the organisation. Once those use cases are delivered, the adoption of the entire data model can follow. If we technically deliver something and it’s not being used by the business and users, it doesn’t bring value, right? For this, we foresee a whole change management process, to make sure that people know how to use the data tools properly, how to share data, how to get insights and answer specific questions. To guarantee the proper adoption of the new tools, we provide training. This way, this new way of working is embraced by everyone.
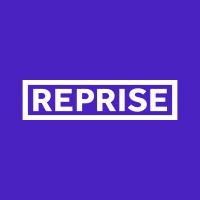
Wondering how you can make sure your business becomes data-driven? Get inspired by Reprise Digital’s customer story.